What is conversion: An explanation for beginners
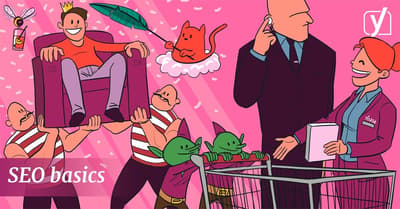
First things first. Conversion isn’t SEO. Conversion is an end of the user journey on your website. It’s not the end, as that customer could come back and start a new part of the journey. Conversion can be improved by good SEO, that much is true if you:
- target the right keywords,
- provide the right site structure, and
- make sure your visitor has the best user experience.
By making sure your visitor is served to his or her best needs, you will provide a warm welcome. You will establish a kind of trust, which will make it easier for that visitor to convert.
Before we dive in, if you want to learn more about conversion and other essential SEO skills, you should check out our All-around SEO training! It doesn’t just tell you about SEO: it makes sure you know how to put these skills into actual practice!!But what is conversion?
The question remains: what is conversion? Conversion is often associated with a sale, but in online marketing, defining conversion just by that is too narrow-minded. Conversion, the way we define it, happens every time a visitor completes a desired action on your website. That could be a click-through to the next page if that is your main goal on a certain page. It could be the subscription to a newsletter. And it could be a visitor buying your product. In short: conversion happens when someone completes the action you want them to complete.
And what is conversion rate?
If 100 persons visit your page, and 10 of them subscribe to your newsletter, your conversion rate is 10%. The conversion rate is also a number you’ll find in Google Analytics, for instance:
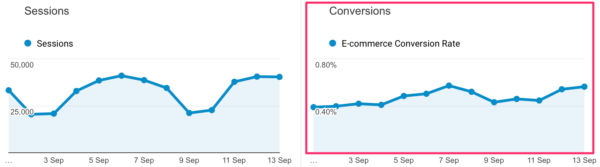
Conversion rate is used to monitor the conversion on a web page, depending on the thing you want to monitor. So, for instance, if you hire our conversion friends at AGConsult, they will (among other things) perform A/B tests on your website and tell you which variant has the highest conversion rate (test winner). You’d better implement that test winner asap, as you will understand :)
How about CRO?
You might even have heard of something called CRO when talking or reading about conversion. CRO is Conversion Rate Optimization. This is the process of optimizing the number of conversions compared to the number of visitors. There are a lot of ways to do this, but again, we’d rather refer you to the conversion experts instead of sharing our basic CRO knowledge. There is just so much to it.
CRO versus SEO
They clash. CRO and SEO clash sometimes. In SEO, we will always tell you to keep the visitor in mind. If you serve the visitor the best way you can, you are optimizing for a brand, for brand loyalty, for recognition in Google, for more and quality traffic. And although the next step might indeed be converting that visitor, there’s no need to shove your products down their throat, really. There is a fine line between serving the visitor and annoying him.
CRO at Yoast
You might think that we, at Yoast, are pretty focused on optimizing that conversion to the max. You see our banners in the plugin, on our website, and indeed, conversion is very important for us. More newsletter subscriptions lead to a larger reach, which leads to more attention for our products, which leads to more reviews, more downloads, more sales. And with the money made, we develop the free plugin and sponsor for instance WordCamps.
Conversion, in any possible way, helps to create sustainable business growth. But trust me, if we wouldn’t keep that SEO, our core business, and our mission (SEO for everyone) in mind, our articles could have six buy buttons. And we could annoy you with a surplus of exit-intent popups and so on. It would upset you, increase bounce rate, trigger you never to come back, and ruin the SEO you so carefully worked on.
So, what is conversion again?
A successful conversion is every instance when a visitor completes a desired action on your website. And still feels good about your brand, your website, and your products, and is likely to come back to your site!
Read more: eCommerce usability: the ultimate guide »
Coming up next!
-
WordCamp Europe 2024
June 13 - 15, 2024 Team Yoast is at Attending, Organizing, Sponsoring, Volunteering WordCamp Europe 2024! Click through to see who will be there, what we will do, and more! See where you can find us next » -
The SEO update by Yoast - April 2024 Edition
30 April 2024 Get expert analysis on the latest SEO news developments with Carolyn Shelby and Alex Moss. Join our upcoming update! 📺️ All Yoast SEO webinars »
I’m totally convinced that the conversion can be improved by good SEO, and that is why it’s important. A very useful article where the most basic is clarified!
Congratulations
Conversation explained well,
Hopefully, it will also help to boost sales and leads as well. And Yoast is also giving a big hand to the internet users for better CRO. Thanks for this awesome piece of writing.
Brilliant post. One successful conversion depends on your website structure, layout, useful information, call to action button and etc. It also depends on brand too. Good SEO can helps generate more conversion. Thank you for this share.
what i consider as a full conversion is when the same customer keeps coming back for what you have to offer and even brings other customer along with him… only good customer support and a great product will do that!
Much more than sales, I see the conversion as “customer loyalty”, that is, to get the same customer to stay engaged by consuming the content made available. This to me is the result of all the effort in SEO and marketing strategy.